Section for Visual Computing
The section for Visual Computing carries out research in image analysis and computer graphics. The goal of image analysis is to extract information from images whereas the goal of computer graphics is concerned with image synthesis. Even though the goals are converse, there is a large overlap in methodology between the two fields.
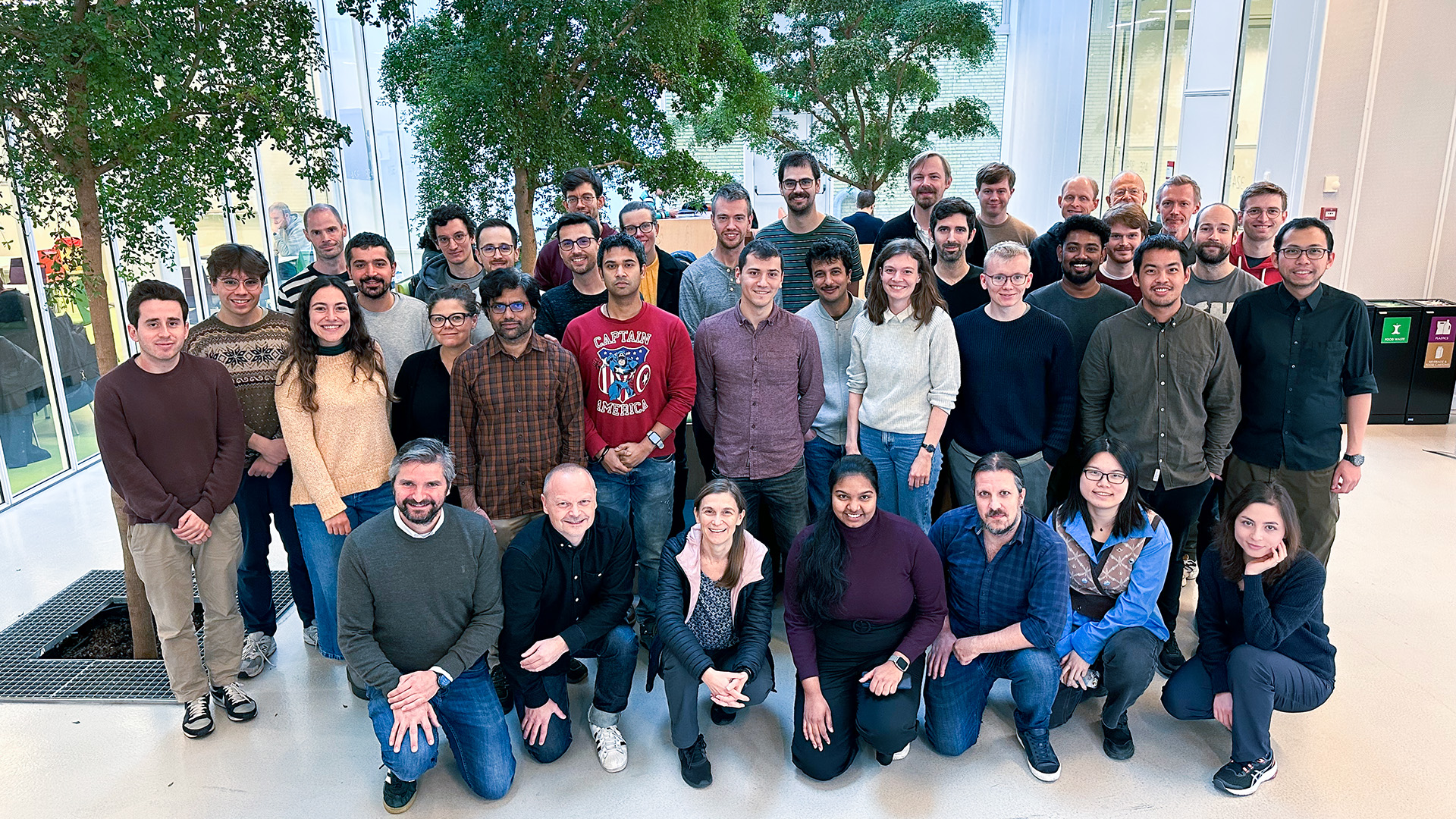
The research at this section is focused on medical image analysis, geometry and appearance modelling, computer vision, multivariate statistics for images and analysis of 3D microstructures. Within these areas, our overarching research aim is to make methodological contributions of broad applicability but motivated by applications and often informed by our collaborations with partners in applied domains. Our contributions have often resulted in innovation, and we are proud of the fact that several start-ups have been founded by former members of the section.